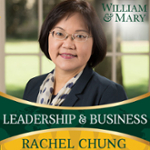
Rachel Chung
Episode 216: February 5, 2024
Myths & Truths About AI
It's been a little over a year now since most of us were introduced to ChatGPT. Since that time, many professionals, businesses, and organizations have worked hard to understand not only ChatGPT, but machine learning and Artificial Intelligence and what it all can do to assist employees, serve customers, and positively affect the bottom line. There's no shortage of information in media coverage regarding Artificial Intelligence, but in the race to embrace AI, it can be challenging to determine what's fact and what's fiction. Our guest today is here to help. Rachel Chung is an award-winning professor at William & Mary's School of Business. Among other things, she teaches business analytics, machine learning, and AI. She joins us today to discuss the myths and truths about Artificial Intelligence.
Podcast (audio)
Rachel Chung: Myths & Truths About AI TRANSCRIPT DOWNLOAD (PDF)
Podcast (platforms)
iTunes | Stitcher | SoundCloud | Amazon Music/Audible | Spotify | Google Podcasts
Show Notes
|
TranscriptRachel ChungDuring the course of a day, you probably use at least a dozen different AI applications, starting with Google Search, right? So, if you search for something online, that algorithm is an AI. Female VoiceFrom William & Mary in Williamsburg, Virginia. This is Leadership & Business, produced by the William & Mary School of Business and its MBA program. Offered in four formats: the full-time, the part-time, the online, and the executive MBA. For more information, visit wm.edu. Ken WhiteWelcome to Leadership & Business, the podcast that brings you the latest and best thinking from today's business leaders from across the world. Sharing strategies, information, and insight that help you become a more effective leader, communicator, and professional. I'm your host, Ken White. Thanks for listening. Well, it's been a little over a year now since most of us were introduced to ChatGPT. Well, since that time, many professionals, businesses, and organizations have worked hard to understand not only ChatGPT but machine learning and artificial intelligence and what it all can do to assist employees, serve customers, and positively affect the bottom line. There's no shortage of information and media coverage regarding artificial intelligence. But in the race to embrace AI, it can be challenging to determine what's fact and what's fiction. Our guest today is here to help. Rachel Chung is an award-winning professor at William & Mary's School of Business. Among other things, she teaches business analytics, machine learning, and AI. She joins us today to discuss the myths and truths about artificial intelligence. Here's our conversation with Professor Rachel Chung. Ken WhiteWell, Rachel, thank you very much for taking time. It's nice to see you. What an interesting topic. Everybody's been talking about AI and ChatGPT, probably for at least the last year. And it's funny how, to me, the knowledge, some know a little, some know a lot. I think most people are sort of in the middle of all of this. But to start off, could you help us define because I think these terms tend to be interchangeable. At least, that's how people tend to use them. What is AI? What is machine learning? And what is ChatGPT? Rachel ChungSo that's a great question. And we hear about AI all in the sudden, in the news all the time. And a lot of people seem to think that AI just kind of started in 2022. But AI actually has been around for decades, not hundreds of years. It's still a relatively new science, but it's been around for probably over 40 years at least. AI stands for artificial intelligence, and intelligence is something that we all have. So people are intelligent, babies are intelligent, even your puppy is very intelligent. And artificial intelligence just means instead of natural intelligence, like what your puppy has. Artificial means that it's something that's artificially made by people. So it's something that we create. And in this case, usually, it's a machine that has somewhat intelligent behavior. I would say that AI is really the scientist's best effort to simulate the brilliance that you and I have. Right. So, we are the true brilliance, and AI is just an approximation of the intelligent behavior that we have. Ken WhiteOh, that's terrific. Machine learning. How do you define that? Rachel ChungSo, machine learning, the key part is learning. So, machine actually is equivalent to the word artificial in AI. So, remember, artificial intelligence is intelligence that's implemented by machines that are artificially made by people. So, machine really just means machine learning is almost like artificial learning, right? So it's a machine that's capable of doing the kind of learning behavior that you and I are able to do. Your puppy can learn as well. A baby can learn. And we can also get a machine to learn from not knowing something very much to being able to perform a task very well. So that learning curve that we're talking about, basically starting with making lots of mistakes and then reducing the number or the quality of the mistakes over time, is the kind of evidence that we want to see in learning. And for machines to learn, we need to be able to see that as well. The machines starting with making a lot of mistakes but making fewer and fewer mistakes over time. Ken WhiteAnd ChatGPT, how do you define that? Rachel ChungSo, ChatGPT is a type of AI, artificial intelligence, and it's an implementation of a large language model. And the large language model it really just means that it's a model that has lots and lots of parameters, and it's used primarily to generate language. Language can be speech or text, written text. And that is a model really is a mathematical model. And all AI models are mathematical models, actually. So these are computed by your computer. So, a machine really is, in this case, a computer. And the computer computes, just like your calculator calculates, the computer computes. So, computation includes doing a lot of math repeatedly. So, it's an automation of all the mathematics that we usually do in school. Ken WhiteAnd you said earlier AI has been around. We might not be aware, but what are some examples of how we've been using this recently and in the past few years? Rachel ChungSo, during the course of a day, you probably use at least a dozen different AI applications, starting with Google Search, right? So, if you search for something online, that algorithm is an AI. If you watch Netflix videos and you see all these recommendations, if you respond to a recommendation by watching something that's being recommended to you, you're using AI. However, if you are recommended a film and you decide not to watch it, you are also helping to train AI. So you're giving some sort of feedback that you don't like this film, you like something else. And that signal is used to train the Netflix AI algorithm to make it smarter and getting to know you a little better. Ken WhiteThat ties into, I look at a story on a log cabin, and then suddenly I'm getting more information on log cabins, for example. Is it similar to that? Rachel ChungExactly. Yeah. So, the algorithm is calculating all these correlations and associations to determine what you like. So when you decide to watch something on log cabin, you are giving positive feedback to the AI machine and to get it to recommend more and more products that are similar. In a way, it's very similar to how you train your puppies to learn something that you want your puppy to do. Ken WhiteInteresting. So there are some myths out there about AI, a lot of misunderstandings, and people just aren't quite sure what's happening. And we've got a couple, I'll say them, and I'd love for you to respond. For example, AI, machine learning, and deep learning are the same things. Rachel ChungSo deep learning and machine learning are very popular types of AI, but AI also include other algorithms that are not deep learning or machine learning. So, as we mentioned earlier, machine learning is a type of learning algorithm and that includes many different kinds of algorithms that learn. And deep learning is what we traditionally call neural networks. And that is just a type of learning algorithms, a type of machine learning algorithms. However, AI also includes other algorithms. For example, recommendation systems is not necessarily deep learning. It can be deep learning, but there are other types of recommendation algorithms that are actually very popular everywhere. It's probably one of the most well-known and well-used algorithm, probably the most successful for businesses. Right? Think about how many businesses actually use recommendation systems. That's probably the number one most popular, wildly used, and we all love our recommendation systems. So, that is something that's not necessarily deep learning. Another one is called reinforcement learning, and that is not deep learning. So reinforcement learning is learning to do things step by step, sequentially. That does not have immediate feedback on whether your action is right or wrong. So that is actually used for AI's to play chess or the go game. And the algorithm that Alphago and a lot of the algorithms that are very successful for winning these chess games are reinforcement learning. So, those are also not deep learning algorithms. Ken WhiteAI systems are only as good as the data they train on. Is that a myth? Rachel ChungSo, data is a very important part of AI. However, the algorithm is also very important. So yes, you need to have high-quality data, but you also need to have algorithm that is appropriate for that data. So both of those components are very important. And these days, there are also many ways to address poor data quality issues or small data issues because there are many, many innovative methods to generate synthetic data, meaning that if you have a small data set, we can duplicate or clone that or simulate to make it a large data set. So basically, making up a lot of fake data for the machine to learn from. The fake data set start look very similar to the real data set. That's actually very popular in both healthcare and finance when data has to be confidential. And so, it is very difficult to train machines using real data. So, these sectors actually are very invested in generating simulated data. Ken WhiteAI systems are inherently unfair. Rachel ChungSo, AI systems, when we talk about AI systems, they are the algorithm and the data. The data are, in fact, generated by the world, right? So you and I, even when we breathe, we are generating data. Every moment that we're alive, we're typing something into the computer, we're speaking to our computer, we're generating data. So, ultimately, data are usually generated by people, not by the computer. The computer is really just learning from the data that's generated by people. So, if there's any sort of bias, it's usually inherent in the data that is coming from the people who generate that data. So, if people are biased, the data is biased. The algorithm, on the other hand, is a set of mathematical models, really, and they are really mathematical equations. I think people are trying very hard not to write biased math algorithms, and ultimately, the machines are really just executing the math for us very efficiently. They really don't have any inherent bias in them, usually. Ken WhiteWe'll continue our discussion with Professor Rachel Chung in just a minute. Our podcast is brought to you by the William & Mary School of Business. The Financial Times, Bloomberg Businessweek, Princeton Review, and US News and World Report have all named the William & Mary MBA program one of the best in the US and the world. If you're thinking about pursuing an MBA, consider one that has world-class faculty, unparalleled student support, and a brand that's highly respected, the William & Mary MBA. Reach out to our admissions team to learn which of our four MBA programs best fits you: the full-time, the part-time, the online, and the executive. Check out the MBA program at William & Mary at wm.edu. Now, back to our conversation with Professor Rachel Chung of the William & Mary School of Business. Ken WhiteAI will make human labor obsolete. Rachel ChungSo that question is very interesting. So there's a lot of fear and a lot of anxiety about job loss due to AI and the news in 2023. We have seen so many headlines and discussions about that. And all the consulting firms have all these numbers, either calculate that they can replace 25% of their workforce. Lots of these different reports have really high numbers estimated for AI replacement. However, today, I just saw an article, a research study coming out of MIT, saying that these are overestimates based on their very detailed interviews and economic analysis. A lot of these tasks because AI can do specific tasks. We don't have general artificial intelligence yet, meaning that we might be able to replace how you write an email, but we cannot replace what you actually do during the day. So, the human actually can do a bunch of different things. AI can only replace a very small set of that. And then, whatever the AI produces, the human still has to inspect it, refine it, edit it, approve it, and so on. So, at the end of the day, you still have to hire a human because the AI can only replace a very small portion of a specific task, and the AI can only do things under supervision. Someone still has to verify if the output is correct or not. Developing and deploying these AI models is still very expensive, and then it's also very expensive to maintain the AI as well. So, so far in the year of 2024, for many, many tasks, it's still way cheaper to hire the human labor than to hire the AI labor. Ken WhiteIt was interesting you mentioned the MIT study. I just read it just this morning in an e-newsletter from the Kellogg School at Northwestern. They looked at the 1950 census, and there were 270 jobs listed in the census. And the question was, how many of those have disappeared because of automation and technology? And it turned out just one. And it was an elevator operator, which I thought was, wow. So, yeah, that overhype again. We hear that so often, don't we? Oh, look out. Here's what's coming. Your job is going to be gone. So it's interesting to hear your take on that. One final myth AI is approaching human intelligence. Rachel ChungSo, AI currently is very good at performing very narrow tasks. For example, AI can recognize an image, label an image. But for you to be able to do complete a task, you might have to recognize something, and then you have to turn around to say something, right? Or maybe you have to go write something down. So, a human usually can perform a number of tasks together in a specific job, whereas AI, right now, we do not have the general intelligence that humans typically have, which means that you can perform a variety of tasks. Current AI technologies can only perform one specific task at a time. So, for example, ChatGPT can write text, but ChatGPT cannot sing. ChatGPT cannot pick up trash from the floor. There are very simple things that we do. ChatGPT cannot walk down the steps and go up, or ChatGPT cannot prevent you from running into the wall. So there are many, many tasks that we do every day that we take for granted that ChatGPT cannot do. Ken WhiteWhat's the future hold? What do you think? Where are we headed with AI? That's a huge question. Rachel ChungWell, the future, I think, is very exciting, with so much attention, and energy, and investment in AI, which is really something I think the AI scientists deserve. AI, the field of AI, went through a long winter when nobody thought that there is a future for AI. Many, many leading AI scientists today, they're still fairly young because it's a young field. And they will tell you that when they were in graduate school and they told people that they were doing AI, most people will tell them that you will not be able to find a job. There's no future because your results are not going to happen. And many people, actually, that I know personally, did not complete their PhDs because it was just way too difficult to actually finish and deliver your AI results. And that, a lot of times was, now we know it was limited by hardware. So, thanks to all the video game players, we now have GPUs. And these GPUs, the graphical processing units that supplement our CPUs, is what enabled AI because the video games need very fast processors, and there was a market for that. And that's why these companies produced GPUs, and they were really produced for video gamers, not for AI. But the AI scientists realized that those units can be used to actually run the AI tasks that they were unable to finish ten years ago. So thanks to all these technological advances, that's why we can enjoy a lot of the AI innovations that we see today. And I think that given all the interest and opportunities for AI, we are going to see leaps and bounds of innovation in the coming years. Ken WhiteThat's our conversation with Professor Rachel Chung, and that's it for this episode of Leadership & Business. Our podcast is brought to you by the William & Mary School of Business, home of the MBA program offered in four formats: the full-time, the part-time, the online, and the executive MBA. Check out the William & Mary MBA program at wm.edu. Thanks to our guest, Rachel Chung, and thanks to you for joining us. I'm Ken White. Wishing you a safe, happy, and productive week ahead. Female VoiceWe'd like to hear from you regarding the podcast. We invite you to share your ideas, questions, and thoughts with us by emailing us at podcast@wm.edu. Thanks for listening to Leadership & Business. |